Digital Threading: How to Extract Maximum Value from Product Data
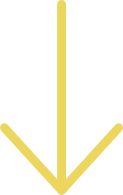
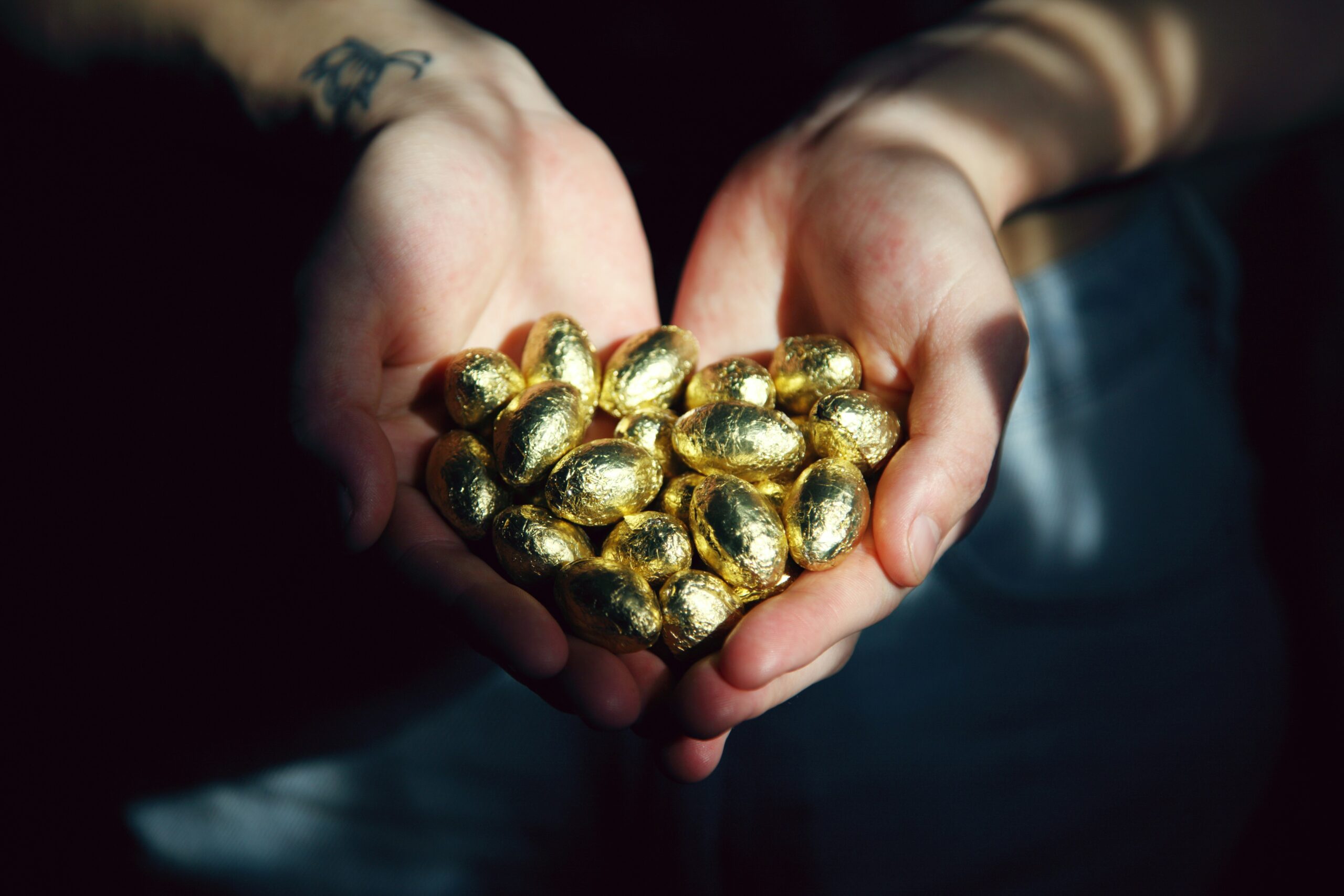
Your product might seem like just… a product. But beneath its surface lies a treasure trove of untapped potential: product data. From purchase patterns to customer reviews, this data holds the key to unlocking valuable insights that can transform your business. But how do you turn this raw data into actionable gold? We’ll explore strategies for collecting, analyzing, and interpreting this information, empowering you to make informed decisions, optimize your product offering, and ultimately, maximize customer satisfaction and business growth.
Digital threading essentially involves the interconnected flow of product data across different stages of the product lifecycle, from design and manufacturing to distribution and beyond. It allows businesses to track and monitor every aspect of their products in real-time, facilitating better collaboration, decision-making, and problem-solving throughout the entire value chain. By leveraging advanced technologies such as IoT sensors, cloud computing, and data analytics, companies can create a digital thread that provides valuable insights and intelligence at every touchpoint.
Understanding Product Data
Product data encompasses a wide range of information related to a particular product or service offered by a company. This data can include various attributes such as size, color, weight, pricing, and more. Essentially, product data provides a comprehensive snapshot of a product’s characteristics, features, and specifications, allowing businesses to effectively communicate its value proposition to customers and stakeholders.
Types of Product Data
There are several types of product data that organizations typically deal with:
- Attributes: These are the specific characteristics or properties of a product, such as dimensions, materials, and functionalities.
- Specifications: This type of data outlines the technical details and requirements of a product, including performance metrics, standards compliance, and compatibility information.
- Usage Data: Usage data refers to information collected about how customers interact with a product, such as frequency of use, user preferences, and product performance over time. Analyzing usage data can provide valuable insights into customer behavior and preferences, guiding product development and marketing strategies.
Sources of Product Data
Product data can be sourced from various channels and systems within an organization, as well as external sources:
- Internal Systems: Enterprise resource planning (ERP) systems, product lifecycle management (PLM) software, and customer relationship management (CRM) platforms are common sources of internal product data.
- Customer Feedback: Customer feedback channels, including surveys, reviews, and support interactions, provide valuable insights into customer preferences, satisfaction levels, and pain points related to product offerings.
- Suppliers: Suppliers and partners often contribute to product data through information about raw materials, components, and manufacturing processes. Collaborating closely with suppliers can ensure the accuracy and completeness of product data throughout the supply chain.
Challenges in Leveraging Product Data
While the potential benefits of product data are immense, organizations often encounter various challenges in effectively leveraging this valuable asset. Overcoming these hurdles is essential for unlocking the full potential of product data and maximizing its impact on business outcomes.
Data Silos and Fragmentation
One of the primary challenges organizations face is the presence of data silos and fragmentation. Product data is often scattered across disparate systems and departments within an organization, making it difficult to access, share, and analyze. This fragmentation inhibits collaboration and hampers the ability to derive meaningful insights from the data, leading to inefficiencies and missed opportunities for optimization.
Poor Data Quality and Inconsistency
Another significant challenge is the issue of poor data quality and inconsistency. Inaccurate, incomplete, or outdated product data can undermine decision-making processes and erode trust in the information being used. Without reliable data, organizations risk making faulty assumptions and strategic missteps that can have far-reaching consequences on product development, marketing campaigns, and customer satisfaction.
Lack of Integration Across Systems
The lack of integration across systems is another hurdle that impedes the effective utilization of product data. Many organizations operate multiple software applications and platforms that house different sets of product data, such as ERP, CRM, and PLM systems. Without seamless integration between these systems, data flows become disjointed, leading to duplication of efforts, data inconsistencies, and missed opportunities for holistic analysis and optimization.
Security and Privacy Concerns
Security and privacy concerns also pose significant challenges in leveraging product data effectively. As organizations collect and store increasing amounts of sensitive information about their products and customers, they become prime targets for cyberattacks and data breaches. Ensuring robust data security measures and compliance with regulatory requirements is essential to safeguarding product data against unauthorized access, theft, and misuse, while also maintaining customer trust and confidence.
Strategies for Effective Data Collection
Effective data collection lays the groundwork for deriving actionable insights and maximizing the value of product data within an organization. By implementing strategic approaches and leveraging appropriate tools and technologies, businesses can streamline their data collection processes and ensure the accuracy, reliability, and integrity of their product data.
Centralizing Data Storage and Management
Centralizing data storage and management is key to overcoming the challenges of data fragmentation and silos. By consolidating product data into a single, unified repository or data warehouse, organizations can facilitate easier access, sharing, and analysis of information across departments and systems. This centralized approach not only improves collaboration and decision-making but also enhances data consistency and integrity.
Implementing Data Governance Policies
Establishing robust data governance policies is essential for maintaining data quality, security, and compliance. Clear guidelines and protocols should be put in place to govern the collection, storage, access, and usage of product data, ensuring that it remains accurate, up-to-date, and relevant. By defining roles and responsibilities, enforcing data standards, and implementing data quality checks, organizations can minimize the risks of errors, inconsistencies, and unauthorized access.
Utilizing Automation Tools for Data Collection
Automation tools can significantly streamline the data collection process, enabling organizations to gather and process product data more efficiently and effectively. From automated data extraction and validation to real-time monitoring and alerts, automation technologies can help reduce manual effort, minimize human error, and improve data accuracy and timeliness. By leveraging artificial intelligence, machine learning, and robotic process automation, businesses can unlock new insights and opportunities from their product data at scale.
Ensuring Compliance with Data Privacy Regulations
Compliance with data privacy regulations is paramount in today’s regulatory landscape, where stringent laws such as the GDPR and CCPA govern the collection, handling, and protection of personal and sensitive data. Organizations must ensure that their data collection practices adhere to applicable regulations and standards, including obtaining proper consent, implementing data encryption and anonymization techniques, and maintaining audit trails for accountability and transparency.
Enhancing Product Data Quality
Ensuring high-quality product data is essential for organizations striving to make informed decisions, drive innovation, and deliver exceptional customer experiences. By implementing robust strategies and processes to enhance data quality, businesses can unlock the full potential of their product data assets and gain a competitive edge in the marketplace.
Data Cleansing and Normalization Techniques
Data cleansing and normalization techniques are critical for improving the accuracy, consistency, and completeness of product data. This involves identifying and correcting errors, inconsistencies, and redundancies within the data, such as misspellings, duplicate entries, and formatting variations. By standardizing data formats, values, and structures, organizations can eliminate data discrepancies and ensure that their product data is reliable and actionable for analysis and decision-making purposes.
Implementing Data Validation Processes
Implementing data validation processes is essential for detecting and preventing errors and anomalies in product data. This involves applying validation rules and checks to verify the integrity, validity, and compliance of data against predefined criteria and business rules. Automated validation tools and algorithms can help flag inconsistencies, outliers, and inaccuracies in real-time, enabling organizations to proactively address issues and maintain data quality standards throughout the data lifecycle.
Regular Data Quality Audits and Maintenance
Regular data quality audits and maintenance are crucial for monitoring and improving the overall health and integrity of product data over time. This involves conducting periodic assessments and reviews of data quality metrics, such as accuracy, completeness, and timeliness, to identify areas for improvement and optimization. By establishing data quality KPIs and benchmarks, organizations can track performance, prioritize corrective actions, and continuously refine their data management processes to ensure ongoing data quality excellence.
Leveraging Advanced Analytics
Predictive analytics involves using historical data, statistical algorithms, and machine learning techniques to forecast future outcomes and behaviors. Machine learning, a subset of artificial intelligence, enables computers to learn from data patterns and make predictions or decisions without explicit programming. These advanced analytics approaches empower organizations to uncover hidden patterns, correlations, and relationships within their product data, enabling them to anticipate market trends, forecast demand, and optimize business processes more effectively.
Applications of Advanced Analytics in Product Data Analysis
Advanced analytics techniques find myriad applications in product data analysis across various industries and use cases. From demand forecasting and inventory optimization to predictive maintenance and customer segmentation, organizations can leverage predictive analytics and machine learning to extract actionable insights and drive value from their product data. These advanced techniques enable businesses to identify opportunities for cost savings, revenue growth, and operational efficiency improvements, ultimately enhancing competitiveness and customer satisfaction.
Case Studies Demonstrating Successful Implementations
Several organizations have successfully implemented advanced analytics solutions to derive tangible business benefits from their product data. For instance, a leading e-commerce retailer used predictive analytics to personalize product recommendations for customers based on their browsing and purchase history, resulting in a significant increase in sales and customer engagement. Similarly, a manufacturing company employed machine learning algorithms to optimize its supply chain operations, reducing inventory costs and improving delivery performance. These case studies underscore the transformative impact of advanced analytics on driving business innovation and success through more intelligent use of product data.
Data Monetization Opportunities
Product data presents a wealth of opportunities for organizations to explore various revenue generation models. From subscription-based services and licensing agreements to pay-per-use models and premium features, businesses can leverage their product data to create valuable offerings that cater to the needs of customers and stakeholders. Whether it’s providing access to exclusive market insights, offering advanced analytics tools, or enabling targeted advertising and sponsorship opportunities, organizations can monetize their product data in ways that align with their business objectives and customer expectations.
Partnering with Third-Party Data Providers
Partnering with third-party data providers offers organizations an additional avenue for monetizing their product data. By collaborating with reputable data brokers, market research firms, or industry consortia, businesses can leverage their product data to enrich existing datasets, enhance data quality and coverage, and create new revenue-sharing opportunities. These partnerships enable organizations to tap into new markets, expand their reach, and unlock additional value from their product data assets through mutually beneficial arrangements.
Monetizing Insights through Data-as-a-Service Offerings
Data-as-a-Service (DaaS) offerings provide organizations with a platform to monetize their product data by delivering valuable insights and intelligence to customers on-demand. By packaging and commercializing their data assets in the form of subscription-based services or pay-per-use APIs, businesses can provide customers with access to real-time analytics, predictive models, and actionable insights that drive business decision-making and innovation. Whether it’s offering industry benchmarking reports, predictive maintenance solutions, or personalized recommendations, DaaS offerings enable organizations to monetize their product data while providing tangible value to customers.
By exploring revenue generation models, partnering with third-party data providers, and offering data-as-a-service offerings, organizations can unlock the full potential of their product data assets and create sustainable sources of revenue in today’s data-driven economy. Whether it’s through subscription-based services, strategic partnerships, or on-demand insights, the opportunities for data monetization are vast, enabling organizations to capitalize on their data assets and drive business growth and innovation in the digital age.
Digital Threading
We began by delving into the intricacies of product data, including its definition, types, and sources, followed by an examination of the challenges organizations face in leveraging product data effectively. We then explored strategies for enhancing data quality, integrating product data into business processes, and unlocking monetization opportunities through advanced analytics and partnerships. It is evident that effectively leveraging product data is essential for organizations seeking to gain a competitive edge, drive innovation, and deliver exceptional customer experiences. By harnessing the power of product data, businesses can make more informed decisions, optimize operations, and create new revenue streams that fuel growth and sustainability in the digital age.
Ready to elevate your brand and transform your vision to digital solutions? Since 2003, StudioLabs has been trusted to help conceive, create, and produce digital products for the world’s most well-known brands to cutting-edge startups. Partner with StudioLabs and create something phenomenal. Let’s chat and experience StudioLabs in action with a complimentary session tailored to your business needs!